By merging the extraordinary capabilities of quantum machines with the expansive uses of artificial intelligence, AI will be able to deliver with more accuracy and efficiency. As one of the most exciting concepts in technology, Quantum machine learning (QML) has the ability to alter how traditional algorithms work and implement the changes to a cloud storage system, along with numerous features that prove its extensive usefulness. This topic will be discussed in further detail as the essay progresses. As of now I will cover the various implementations of quantum machine learning, its cloud based applications, traditional algorithms, real life implementations and much more.
What Is Quantum Machine Learning?
The basics of Quantum Machine Learning (QML) consists of blending the concepts of machine learning and quantum physics. Because a quantum computer uses qubits instead of classical computers that function on binary bits (0s and 1s) like superposition and entanglement, many states can be processed simultaneously. As a result, an unreasonable amount of data is processed in record breaking time by quantum computers. There are also ways of extracting information through patterns with the aid of algorithms.
The machine learning process consists of first training the algorithms with data then extracting information from calculations or patterns. The powerful QML quantum machine boasts is being put to use to speed up complicated calculations, optimize existing algorithms, and even solve problems that have never been solved before.
How Does Quantum Machine Learning Work?
Quantum machine learning operates by encoding data into quantum states and using quantum circuits to process this information. The primary goal is to exploit quantum phenomena like superposition and entanglement to:
- Speed Up Computation: Quantum computers can perform parallel computations, drastically reducing the time required for tasks like optimization and matrix operations.
- Enhance Accuracy: Quantum models can handle high-dimensional data and intricate patterns, potentially leading to more precise predictions.
- Enable New Algorithms: Quantum mechanics introduces unique capabilities, allowing the creation of novel algorithms that classical systems cannot replicate.
For example, variational quantum circuits are used in QML to optimize parameters in quantum models. These circuits can be trained using classical optimization methods, bridging the gap between classical and quantum computing.
Applications of Quantum Machine Learning
Quantum machine learning has far-reaching applications across various industries. Here are some key sectors where QML is making a significant impact:
1. Finance
- Portfolio Optimization: QML can analyze vast datasets to optimize investment strategies, balancing risk and return more efficiently.
- Fraud Detection: By identifying subtle anomalies in large financial datasets, QML enhances fraud detection mechanisms.
2. Healthcare
- Drug Discovery: QML accelerates molecular simulations, enabling researchers to identify promising drug candidates faster.
- Personalized Medicine: Quantum-powered algorithms analyze genetic data to tailor treatments for individuals.
3. Logistics
- Route Optimization: QML can optimize supply chain logistics, reducing costs and improving efficiency.
- Demand Forecasting: By analyzing historical data, QML predicts future demand with greater accuracy.
4. Artificial Intelligence
- Sentiment Analysis: Advanced quantum-inspired models will enhance NLP applications like voice translation and sentiment classification.
- Training: Tasks in reinforcement learning, particularly robotics and game AI, benefit from quantum-enhanced training.
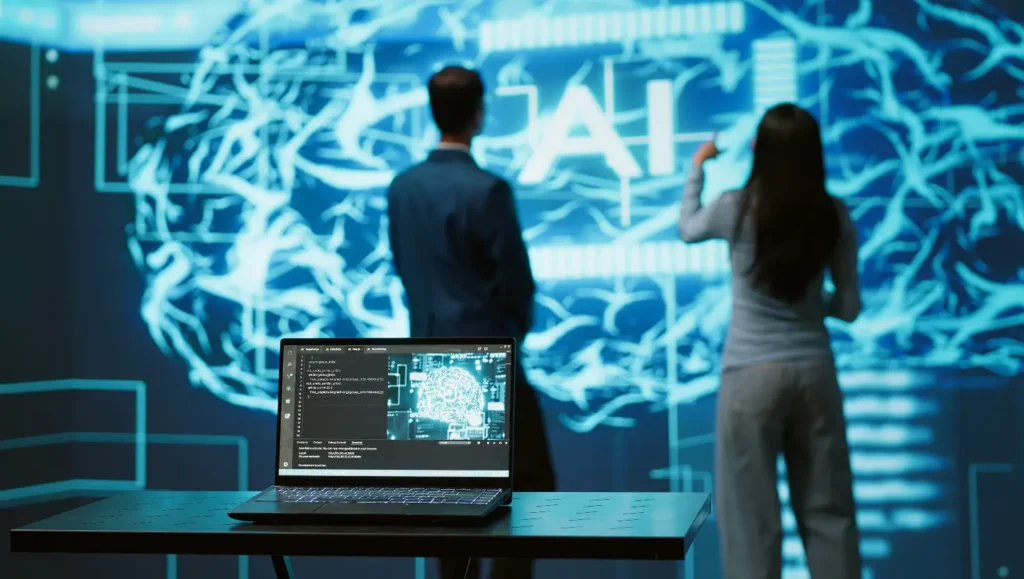
The Role of Cloud-Based Quantum Machine Learning
By enabling remote access to quantum architecture and emulators, cloud quantum machine learning is making quantum computing more accessible to all. In the past, affordable quantum computers could only be employed by big research institutions because of their costs and complications. However, now, with the introduction of cloud quantum machine learning, the innovation can be introduced at any level.
Advantages of Cloud-Based Quantum Machine Learning Software:
- Accessibility: Researchers, businesses, and enthusiasts can experiment with quantum algorithms without owning expensive hardware.
- Scalability: Cloud platforms offer scalable resources, allowing users to test and deploy QML models of varying complexities.
- Integration: Many platforms integrate seamlessly with classical machine learning tools, enabling hybrid quantum-classical workflows.
Notable Cloud-Based Quantum Machine Learning Solutions:
- IBM Quantum: implements the Qiskit framework to create and run quantum algorithms. IBM provides cloud services to quantum devices of up to 127 qubits
- Google Quantum AI: Uses its NISQ processors and simulators for testing quantum algorithms.
- Amazon Braket: supplies a complete setting for constructing, refining, and executing quantum algorithms on different computing devices.
- Azure Quantum: Integrates several quantum hardware components with its cloud system and support Qiskit, Cirq, and Microsoft’s Q#.
Quantum Machine Learning Algorithms
Several algorithms exemplify the synergy between quantum mechanics and machine learning. Here are some notable ones:
1. Quantum Support Vector Machines (QSVMs):
- Used for classification tasks, QSVMs leverage quantum kernels to handle high-dimensional data effectively.
2. Quantum Neural Networks (QNNs):
- By encoding data into quantum states, QNNs optimize weight parameters using quantum circuits, enabling faster convergence.
3. Quantum Principal Component Analysis (QPCA):
- Accelerates dimensionality reduction by extracting principal components from large datasets exponentially faster than classical methods.
4. Quantum Boltzmann Machines (QBMs):
- Enhance generative modeling tasks by utilizing quantum annealing for efficient training.
Challenges in Quantum Machine Learning
While QML holds immense promise, it’s essential to address the challenges that come with this emerging field:
1. Hardware Limitations:
- Current quantum computers are in the Noisy Intermediate-Scale Quantum (NISQ) era, meaning they are prone to errors and have limited qubit counts
2. Algorithm Development:
- Creating useful quantum algorithms is highly complex and requires knowledge of both quantum physics and machine learning, which is challenging.
3. Data Encoding:
- Translating classical data into quantum states is complex and can limit the efficiency of quantum algorithms.
4. Cost and Accessibility:
- While cloud-based solutions reduce barriers, quantum computing resources remain costly for extensive experimentation.
Real-World Examples of Quantum Machine Learning
Several organizations are already leveraging quantum machine learning to achieve breakthroughs:
Volkswagen:
- Using quantum algorithms to optimize traffic flow in urban areas, reducing congestion and emissions.
Deloitte:
- Exploring QML for financial risk modeling and fraud detection, enabling more robust decision-making.
Biogen:
- Partnering with quantum computing firms to accelerate drug discovery and molecular simulations.
NASA:
- Investigating QML for optimizing satellite scheduling and space mission planning.
The Future of Quantum Machine Learning
Quantum machine learning is poised to become a cornerstone of technological progress. As quantum hardware matures and more robust algorithms are developed, we can expect:
1. Enhanced Cloud Solutions:
- Cloud-based quantum machine learning software will offer even greater integration with classical systems, enabling seamless hybrid workflows.
2. Industry Adoption:
- Sectors like finance, healthcare, and logistics will increasingly adopt QML for competitive advantages.
3. Educational Resources:
- As awareness grows, more accessible training programs will empower the next generation of quantum scientists and engineers.
4. Breakthrough Applications:
- From climate modeling to cryptography, QML will unlock new possibilities across disciplines.
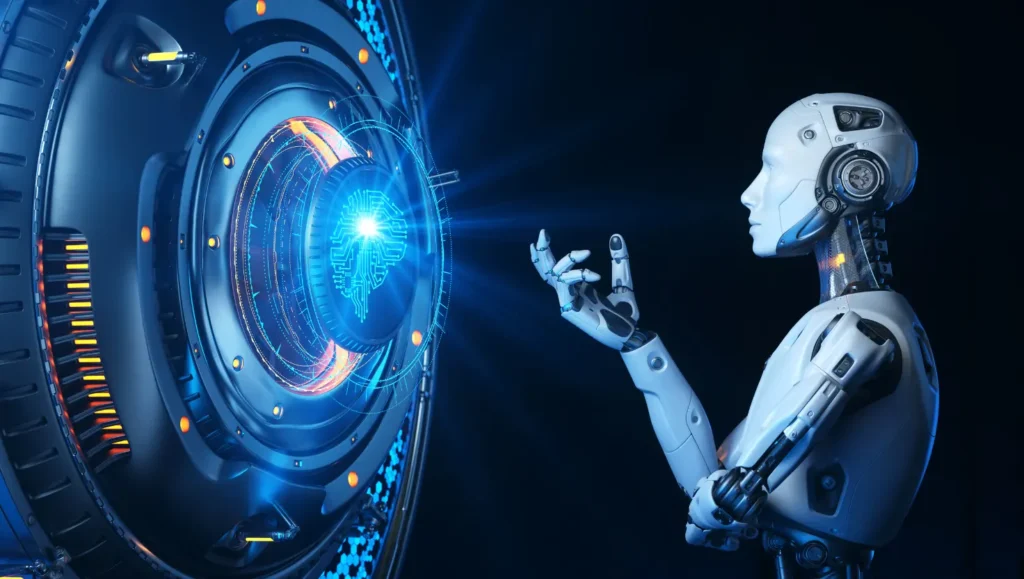
Conclusion:
The introduction of quantum machine learning acts as a leap into the future by blurring the boundaries of human made computers and artificial intelligence. Now, with the introduction of cloud enabled quantum machine learning solutions, this technology is easily available to all industries, which in turn will make astounding changes to business models. With this, there is very likely a revolution in the field of data processing and physical interaction with computers. Quantum machine learning will redefine all of these concepts.
The adoption of quantum machine learning today is no longer about coping with technological changes; it is about anticipating what is to come. Regardless if one is a businessman, researcher, or a fan, the time is ripe to appreciate what can be achieved with QML technology.
FAQs:
1. How does quantum computing enhance machine learning?
Machine learning is enhanced significantly with the implementation of quantum computing due to:
- Neural networks requiring critical matrix calculations to be done faster.
- Quantum parallelism allowing the exploration of large data spaces at the same time.
- Classical systems struggling with optimization problems being solved successfully
2. What are some real-world applications of quantum machine learning?
The various industries that quantum machine learning can be implemented in includes:
- Drug creation and personalized medicine within healthcare.
- Fraud detection and portfolio optimization in finance.
- Managing supply chains logistics.
- Simulating the interactions of molecules in quantum chemistry.
4. What is cloud-based quantum machine learning?
Cloud quantum machine learning goes hand in hand with quantum machine learning and involves accessing software and computing resources on the cloud. This is done in order to develop algorithms for quantum machine learning and execute them. These solutions do not require owning any kind of quantum hardware technology, making it easier to utilize these types of technologies.
5. What are the benefits of cloud-based quantum machine learning software?
- Cost savings by avoiding hardware investments.
- Accessibility for researchers and developers worldwide.
- Scalability to test and execute large quantum algorithms.
- Integration with existing machine learning workflows.
6. How does quantum machine learning differ from classical machine learning?
Unlike classical machine learning approaches, quantum machine learning has the unique advantage of utilizing qubits, which allow for parallel computation, meaning, quantum machine learning can access and run numerous datasets at the same time. On the other hand, classical machine learning depends on binary bits, making it inefficient on certain tasks.
7. Which sectors are expected to benefit the most within QML?
Healthcare, finance, logistics, energy, and quantum chemistry are among the industries that could gain the most due to their broad scope for undertaken complex computations and optimizations.